The transformative power of Large Language Models (LLMs) in the professional landscape is undeniable. In about a year, these tools have proliferated throughout the knowledge worker world, with almost every company trying to determine how to use this new technology in their product offerings. Big and small companies are exploring ways to harness this technology for their products. The versatility of these systems suggests that their influence will be far-reaching. But what impact has it actually had on you?
A closer look reveals a disparity in how professionals integrate these tools into their daily tasks. While some have seamlessly embedded them, reaping efficiency benefits, most others have yet to find that it makes much of an impact. Surveys, including my own, indicate a pattern: individuals are intrigued by the capabilities of these tools and experiment with them but often stop short of making them a staple in their workflow.
I have mixed feelings about this reality. On the one hand, I understand the hustle of our daily grind making the adoption of new tools difficult. I also believe there is a responsibility for tech firms to craft tools that seamlessly blend into our professional lives. On the other hand, it concerns me. The efficiency gains I've witnessed through thoughtful utilization are monumental, and those who adeptly weave these advancements into their daily lives are redefining effectiveness.
We, as humans, are not equipped to comprehend exponential growth fully. This cognitive gap is what initially obscured the rapid escalation of a localized virus in Asia into a global health crisis.  It is also why we struggle to recognize how valuable it is to save money when we are young, allowing compound interest to do most of the work. If these technologies do indeed catalyze exponential productivity—as I'm convinced they do—early adopters stand to amplify their efficacy tenfold. Conversely, those who hesitate may find themselves irretrievably behind in this accelerating landscape.
When it comes to embedding these technologies into our routines, I advocate for a proactive stance. In this, I encourage an experimental mindset, finding a way to automate the aspects of one’s job that they find dull while beginning to capture the efficiency gains. My vision is a future where human brain power is reserved for tasks that demand our innate gifts of contextual insight, critical analysis, and empathy.
If you choose to stop reading here, I implore you to at least entertain the prospect of these innovations. Should you venture further, this article will guide you in cultivating a growth mindset amidst these technological tides. You'll emerge with a fresh perspective on navigating these shifts, hopefully setting you on a trajectory where the most fulfilling facets of your work are those you enjoy the most.
Two factors contributing to the lack of adoption
Process changes are like any other habit formation; they are time-consuming and require significant repetitions. The rewards of adopting a new habit play a pivotal role in its longevity. If a tool or process significantly enhances one's work, it's more likely to become a mainstay after a few iterations. However, pinpointing this high-value use case with digital tools, especially Large Language Models (LLMs), can be difficult. Two primary factors impede sustained engagement with tools like ChatGPT: the Fresh Start Effect and the dilemma of Choice Overload.
Life's significant transitions offer fertile ground for new habit formation. This phenomenon, coined the Fresh Start Effect by Katie Milkman, underscores the power of new beginnings in driving behavioral change. Starting afresh can diminish the pull of old habits, paving the way for new ones. However, this effect can be a double-edged sword in the digital realm. Most digital platforms aim for continuity, ensuring users pick up where they left off. In opposition, LLMs present users with a blank slate, emphasizing boundless possibilities but devoid of any prior context.
Imagine working on a report, taking a detour to an LLM tool to punch up some of the content, and being greeted with an empty canvas. Without a solid habit to default into, you are left wondering what exactly you should ask or what the best prompt to complete your task could be. The ease of reverting to familiar processes becomes tempting, with vague promises of revisiting the tool another day. The blank canvas can also disorient, leading users to diverge from their original task, lured by the tool's myriad possibilities.
Compounding this is the paralysis of Choice Overload. Faced with a plethora of options, individuals commonly freeze, unable to make a decision. How does one decide where to begin amidst this sea of possibilities?
More often than not, choices are made based on recency rather than efficacy. Given that optimal interactions with LLMs often involve a degree of experimentation, it's unsurprising that many settle for less-than-ideal outcomes. The sheer volume of choices, coupled with the constant reset with each interaction, presents formidable barriers to the habitual use of these tools. Until these tools evolve to address these challenges, users must be proactive to be able to derive consistent value.
A Framework for LLM habit formation
Intertwining the powerful capabilities of new generative AI systems into one’s workflow is akin to embarking on any transformation journey. Just as one musters the discipline to hit the gym or set aside savings, integrating LLMs into one's routine demands a strategic approach rooted in behavioral science principles. To navigate this journey, I propose a structured five-step methodology that identifies tasks ripe for LLM enhancement and fosters a sustainable habit around its use.
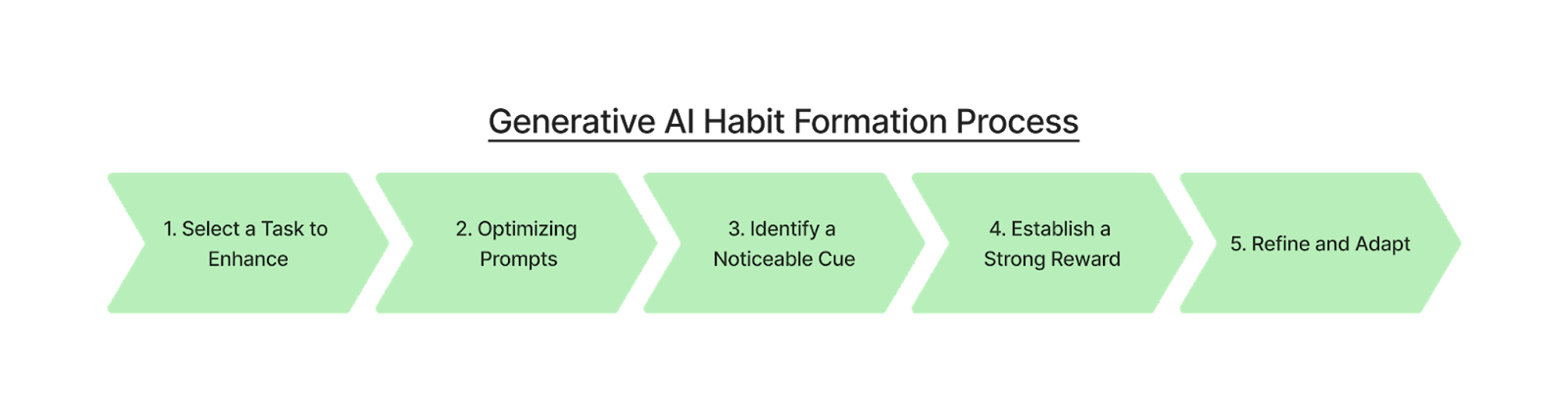
The genesis of any habit lies in pinpointing specific scenarios where the tool's advantages are palpable. Once such opportunities are discerned, the subsequent phase involves a period of experimentation. This phase is crucial to determine the optimal prompts that harness the tool's potential for the identified task. With a refined strategy, the stage is set for habit cultivation. Drawing inspiration from James Clear's Habit Loop, the process can be distilled into three core components: a compelling cue that triggers engagement, a defined action executed within the LLM platform, and a tangible reward that reinforces the behavior. However, it's essential to recognize that this loop isn't static. As with any evolving tool, one must remain agile, periodically revisiting and refining their prompts and strategies to stay aligned with the ever-evolving capabilities of LLMs.
Identifying Tasks Most Suited for Enhancement with LLMs
A unique blend of routine tasks and sporadic responsibilities characterizes every profession. Given this diversity, there isn't a one-size-fits-all blueprint for seamlessly integrating LLMs into one's daily grind. The key, instead, lies in pinpointing specific activities where these models can add significant value. A great place is with tasks centered around content creation or modification - one of the most powerful aspects of these new systems. With this knowledge, think about some of the areas of your job that stand to benefit from the addition of these tools.
Drawing from my research experiences, I've discovered several tasks that have been profoundly transformed through the power of generative AI. In the ensuing discussion, I'll delve into a series of task examples that have not only revolutionized my workflow but also hold the potential to resonate with a broad spectrum of knowledge workers.
Brainstorming Ideas - Generating project opportunities
Determining what types of work could be done to generate value for stakeholders comes with experience. With some probing, AI can begin to make up for a lack of ideas by generating diverse starting points for a project based on provided parameters. This can foster creativity and innovation by offering unexpected ideas. A simple prompt can begin generating ideas. Once there is traction in one or two areas, further discussion can continue to create ideas and the types of questions to ask to uncover more information.
Example Conversation Using ChatGPT
Prompt:
You are an experienced applied behavioral scientist who is now working at a bank. It is time for you to choose a few places to look for projects to help users achieve better outcomes. Create a list of 3 places that might be good opportunities for projects to be created and who would likely be the best contact to reach out to discuss.
Response:
Online Banking Platform: A key area to explore is the online banking platform, as this is often a primary method of interaction between customers and the bank. Improvements in this area could lead to better user experiences, more successful transactions, and increased customer loyalty. A suitable person to connect with would be the Head of Digital Products, as they would have a comprehensive understanding of the existing online services and potential areas for improvement.
Financial Education Programs: Providing educational resources about financial management can be invaluable to customers, especially those with limited financial literacy. A project here could focus on creating user-friendly tools, interactive tutorials, or workshops to increase customer knowledge. The Head of Customer Engagement or Director of Community Outreach would be the most appropriate contacts for this project.
Customer Support Services: Addressing any gaps in the bank's customer support services could significantly enhance customer satisfaction. This could be done by examining the current system for handling customer queries or complaints and devising behavioral strategies to improve this process. The Head of Customer Service is the best person to approach regarding projects related to customer support.
Rewriting Content - Messages based on behavioral techniques
Utilizing behavioral techniques in notifications or email headers is a common first step when applying behavioral science. Using different techniques, Generative AI can create compelling and effective messages across various topics. Providing a message and a few behavioral techniques will yield multiple messages that can be deployed at different times or, even better, in an A/B test. This shows the great potential of AI to rewrite all sorts of content.
Example Conversation Using ChatGPT
Prompt:
Take the following message, "Remember to complete a workout today" and create a list of rewritten versions based on the following behavioral techniques: Social Norms, Loss Aversion, and Commitment Bias.
Response:
Sure, here are different ways to rephrase the message, "Remember to complete a workout today", based on the behavioral techniques you mentioned:
Social Norms:
"Don't forget, many successful people incorporate a workout into their daily routine."
"Join thousands who are choosing to get fit today, remember to work out."
"Stay on par with your peers - complete your workout today."
Loss Aversion:
"Don't let your progress slip away, remember to work out today."
"Avoid missing out on your fitness goals, make sure you workout today."
"Preserve your health benefits - don't forget to workout today."
Commitment Bias:
"Stick to your commitment to health, remember to workout today."
"Remember your commitment to fitness, don't forget to workout today."
"Stay true to your promise to yourself - complete your workout today."
Identification of Information Sources - Findings Reports
Applied researchers commonly must rapidly bring themselves up to speed on new topics. At least once a week, I must quickly understand a new concept so that I can determine how to apply it to the work that I am doing. I have found that BARD, Google’s LLM, is especially good at these sorts of tasks since it can search the internet quickly. Here, I asked it to find a few reports and provide me with summaries. You can see that it does a fair job with my instructions, identifying three relevant reports. This same type of task can be repeated for all sorts of information requests.
Example Conversation Using BARD
Prompt:
Identify and write a one-paragraph summary of the top three reports describing how behavioral science is applied in organizations.
Response:
Top 3 Reports Describing How Behavioral Science is Applied in Organizations
1. The Current State of Behavioral Science Teams and Their Techniques by Connor Joyce (2023)
This report presents the findings of a survey of 324 behavioral science teams from around the world. The survey found that the most common applications of behavioral science in organizations are to:
Increase employee engagement and productivity
Improve customer satisfaction and loyalty
Reduce costs and waste
Promote health and well-being
Encourage sustainable behavior
(Truncated for brevity)
Consider these examples as a springboard to explore how they might dovetail with your workflow. Ideally, one of these tasks will resonate, serving as a catalyst for habit formation, which can then be transferred to other facets of your work. If none stand out immediately, continue your quest for tasks that revolve around consistent content creation or modification. Regardless of whether it is one of the suggestions or one that you discover yourself, it's imperative to crystallize the desired outcome, as this sets the stage for the subsequent steps.
Optimizing Prompts for Maximum Value
With one or more tasks identified, the next step is formulating the best prompt to use this technology. A common pitfall with these tools is the complacency that arises from receiving somewhat satisfactory results from mediocre prompts. An excellent prompt can give you an outstanding output; finding something better is worth the investment.
The art of tailoring prompts to align with specific needs is called 'prompt engineering.' At its core, it involves experimenting with diverse prompts to discern which output resonates most with what you desire. It is as simple as testing different prompts to see which output best matches your use case. Yet, it is as nuanced as drastically changing one or more words to change the output. Thus, you should time-box prompt engineering efforts to 20 to 30 minutes, striking a balance between refining the prompt and not getting mired in detail. A pragmatic strategy to enhance a prompt is to pinpoint gaps or desired changes in the output and incorporate them explicitly in the subsequent prompt iteration. However, it's important to note that GPT tends to become less responsive to instructions after around 5 explicit commands.
An alternative perspective to prompt engineering is envisioning the LLM as a novice intern. As a newcomer requires context and orientation, the LLM benefits from a comprehensive backdrop before executing a task. Techniques like role-playing, where you instruct the tool to adopt a specific persona before responding, can be instrumental. Similarly, the 'multi-shot' approach, where you provide illustrative examples of the desired output, proves invaluable for consistent, distinctive results. The guiding principle is to furnish ample context and guidance within a reasonable limit—typically a few concise paragraphs—beyond which the complexity might be counterproductive.
Cultivating New Habits with LLMs
Having a refined prompt for your chosen tasks, the next phase is to seamlessly weave them into your daily routine, drawing inspiration from the principles of habit science. The third and fourth steps of the Generative AI Habit Formation Process revolve around identifying a 'Cue' to signal the opportune moment for LLM deployment and establishing a reward mechanism to reinforce its recurrent use. While I am leveraging the Habit Loop framework for this illustration, feel free to adapt any habit-building methodology that resonates with you.
It's essential to acknowledge that cultivating new habits is a gradual process. However, this journey can be accelerated by curating an environment conducive to habit formation. The initial step is to institute a 'Cue'—a reminder prompting you to harness the capabilities of generative AI for task execution. Ideally, the selected task should be so intrinsically linked to your role that its mere initiation triggers an automatic response, prompting you to engage with the LLM.
However, relying solely on memory might not always suffice. In such scenarios, external reminders, be it tech-driven or tangible cues, can bridge the gap. A daily calendar alert or a strategically placed sticky note could serve as an effective nudge. The key is to innovate—identify the preliminary steps of your chosen task and devise a mechanism that momentarily disrupts your routine, reminding you of your newfound approach. Experimentation is pivotal in discerning what aligns with your workflow.
Subsequent stages in the Habit Loop—craving and action—were addressed by selecting a pertinent task and crafting an effective prompt. The culmination of this loop is the reward phase. Recognizing the efficiency gains alone should be a motivating factor because the task is integral to your role. If the outcome feels lackluster, it might indicate either an ill-chosen task or an ineffectual prompt. If you believe you have a solid prompt and desire a stronger sense of reward, consider the tangible benefits accrued—be it time saved or enhanced output quality. The overarching objective is to conclude each iteration with a sense of accomplishment, reinforcing the adoption of this transformative process.
Refine and adapt
Having established a strong 'Cue' to initiate the process, a genuine aspiration to elevate workplace efficiency, a valuable prompt to streamline tasks, and a gratifying sense of achievement, you're poised to cultivate a transformative habit. Commit to this approach for a few weeks and gauge its success. Should you encounter challenges, review each phase of this methodology and finetune as needed.
However, it's crucial to recognize that even a seemingly perfect habit formed in this space is susceptible to the dynamic nature of our work environment and technological advancements. Hence, the final phase emphasizes perpetual refinement and adaptability. This journey transcends the quest for an optimal solution; it's about harnessing efficiency gains and channeling a fraction of those benefits toward continual process enhancement. Embracing this progressive mindset ensures you remain at the forefront of innovation, safeguarding against unforeseen technological shifts.
Even when the process is going well, I advocate for a monthly review of the tasks and corresponding prompts you enhance. Explore fresh avenues to leverage these technologies and devise superior integration strategies. Discern patterns in your interactions—identify elements that resonate and those that don't. Endeavor to transfer the aspects of positive experiences to less favorable interactions. Whenever the LLM's output appears suboptimal, remember its malleability. With the right prompt, most challenges can be surmounted.
Generative AI, while potent, only realizes its full potential when seamlessly woven into your workflow. Remain adaptable in the face of this change by methodically integrating it into your routine. Should uncertainties arise, circle back to the structured habit formation approach outlined in this piece, recalibrating tasks, prompts, and reinforcing the incentive to re-engage. Doing so will keep you in control of your job and ahead of others who resist this new technology.